The rapid evolution of artificial intelligence (AI) has transformed industries, reshaped economies, and redefined human interaction. By 2030, AI systems are no longer mere tools—they are collaborators, decision-makers, and integral components of societal infrastructure. Yet, as their influence grows, so do the ethical dilemmas they pose. From perpetuating systemic biases to eroding personal privacy and evading accountability, AI’s darker implications demand urgent attention.
The year 2030 marks a turning point. After decades of reactive measures, the global community has shifted toward proactive, systemic solutions to ensure AI aligns with human values. This blog post explores how cutting-edge advancements in algorithmic fairness, privacy-preserving frameworks, and accountability mechanisms are addressing these challenges, creating a blueprint for ethical AI that benefits all of humanity.
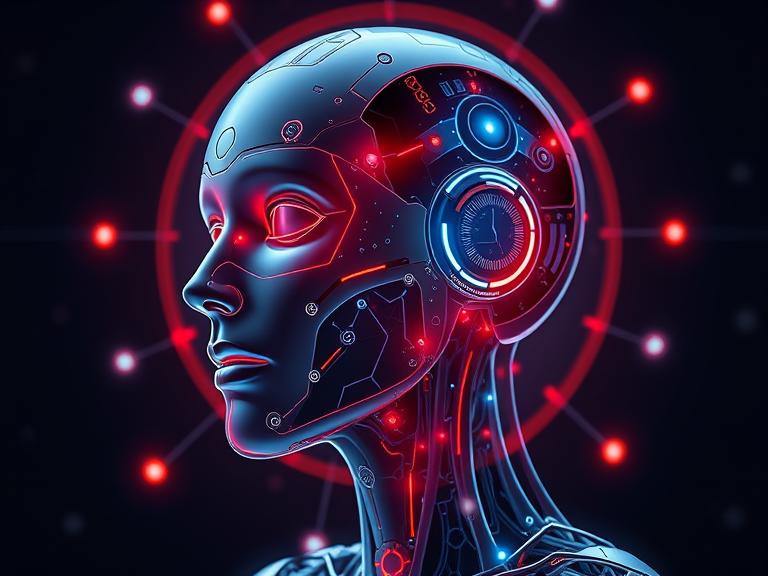
1. Tackling Algorithmic Bias: From Detection to Prevention
The Legacy of Bias in AI
Historically, AI systems have mirrored—and often amplified—human prejudices. Facial recognition software misidentified people of color, hiring algorithms favored male candidates, and predictive policing tools targeted marginalized communities. These issues stemmed from flawed datasets, homogeneous development teams, and a lack of transparency in model design.
By 2030, the narrative is changing. The AI community recognizes that bias isn’t a glitch but a structural problem requiring end-to-end solutions.
Advances in Bias Detection and Mitigation
- Synthetic Data Generation: To combat biased training data, AI developers now use synthetic datasets engineered to represent diverse demographics. For instance, tools like FairSynth generate artificial data points that fill gaps in real-world data, ensuring models aren’t skewed toward dominant groups.
- Explainable AI (XAI): Transparency is no longer optional. Regulatory frameworks mandate that AI systems provide interpretable explanations for their decisions. Techniques like neural symbolic integration allow models to “show their work,” revealing how factors like race or gender influence outcomes.
- Real-Time Bias Correction: Modern AI systems employ “self-auditing” algorithms that continuously scan for discriminatory patterns. If a loan approval model starts favoring certain ZIP codes, it triggers an automatic recalibration.
Case Study: Bias-Free Hiring in 2030
Consider TalentMatch, an AI recruitment platform used by Fortune 500 companies. Unlike its predecessors, TalentMatch uses synthetic data to simulate candidates from underrepresented backgrounds and employs XAI to justify each hiring recommendation. Its success rate in diversifying workforces has reduced employee turnover by 30%, proving ethical AI is also good for business.
2. Reinventing Data Privacy Frameworks: Beyond Encryption
The Privacy Paradox
AI’s hunger for data has long clashed with individual privacy rights. By 2030, however, the rise of privacy-first AI has reconciled this tension. Innovations in decentralized systems and regulatory rigor ensure data protection without stifling innovation.
Key Innovations in Data Privacy
- Federated Learning: AI models are now trained across distributed devices without raw data ever leaving users’ hands. For example, a healthcare AI diagnosing rare diseases learns from millions of smartphones while keeping personal health records local.
- Homomorphic Encryption: Data remains encrypted even during processing. Banks use this to analyze spending habits without exposing transactions, enabling personalized services without compromising privacy.
- Data Ownership Platforms: Blockchain-based systems like DataVault let individuals monetize their data selectively. Users approve specific data streams for specific uses—say, sharing fitness data with a research institute but not advertisers.
Regulatory Landscapes in 2030
The Global Data Privacy Accord (GDPA), ratified in 2027, standardizes privacy laws across 150+ nations. It enforces strict penalties for noncompliance and bans “dark patterns” that trick users into sharing data. Under the GDPA, AI systems must undergo Privacy Impact Assessments (PIAs) before deployment, evaluating risks like re-identification of anonymized data.
Case Study: Healthcare AI with Privacy by Design
In 2030, MediGuard, an AI diagnosing cancer from medical scans, operates under federated learning. Hospitals collaborate to improve its accuracy without sharing patient records. Homomorphic encryption ensures even the AI developer can’t access sensitive data. Result? A 40% faster diagnosis rate with zero privacy breaches.
3. Ensuring Accountability: Who’s Responsible When AI Fails?
The Accountability Gap
Early AI systems existed in a legal gray area. When a self-driving car caused an accident or a diagnostic bot missed a tumor, victims had little recourse. By 2030, accountability is embedded into AI’s lifecycle through stringent audits, certifications, and liability laws.
Mechanisms for AI Accountability
- Mandatory Audits: Independent third parties, like the International AI Ethics Board (IAIEB), conduct annual audits of high-risk AI systems. Auditors review training data, decision-making processes, and incident logs.
- Certification Programs: Similar to UL marks for electronics, the Ethical AI Seal is awarded to systems meeting safety, fairness, and transparency benchmarks. Consumers increasingly demand this certification, driving industry-wide compliance.
- Liability Insurance: Companies using AI must now carry AI Liability Coverage, ensuring victims receive compensation for harms caused by algorithmic errors.
Legal Frameworks in 2030
The EU AI Liability Directive (2029) sets a precedent: developers, deployers, and users share responsibility for AI outcomes. If a biased hiring algorithm rejects a qualified candidate, all three parties face fines proportional to their role in the harm.
Case Study: Autonomous Vehicles and Clear Liability
In 2028, an autonomous truck caused a fatal collision due to a sensor malfunction. Under updated liability laws, the manufacturer (for defective hardware), the AI developer (for flawed object recognition), and the logistics company (for inadequate maintenance) shared penalties. This case spurred industry-wide safety protocols, reducing accidents by 60% by 2030.
4. The Interplay of Bias, Privacy, and Accountability
Ethical AI isn’t about solving one issue at a time—it’s about balancing competing priorities. For instance, mitigating bias might require analyzing sensitive demographic data, which risks privacy violations. Conversely, strict privacy measures can hinder accountability by limiting access to data logs.
Integrated Solutions in 2030
- Privacy-Preserving Bias Checks: Techniques like secure multi-party computation allow auditors to detect bias in AI models without accessing raw data.
- Decentralized Accountability Networks: Blockchain ledgers track AI decisions immutably, creating an audit trail while encrypting personal details.
5. The Road Ahead: Challenges and Opportunities
Despite progress, hurdles remain. Smaller nations struggle to enforce GDPA standards, and “ethics washing” persists among firms that prioritize PR over genuine compliance. Moreover, the rise of quantum computing threatens to crack today’s encryption methods, necessitating constant innovation.
Yet, the momentum is undeniable. Grassroots movements like AI for the People pressure corporations to adopt ethical practices, while global collaborations like the UN AI Ethics Council foster cross-border cooperation.
Conclusion
By 2030, ethical AI is no longer an aspirational goal—it’s a measurable standard. Through synthetic data, federated learning, and robust accountability frameworks, AI systems have become fairer, safer, and more transparent. However, this progress hinges on sustained vigilance. Policymakers, developers, and users must collaborate to update standards as technology evolves.
The lesson is clear: Ethics cannot be an afterthought. It must be the foundation upon which AI is built.