Introduction: The AI Revolution and Its Dual Edges
Artificial Intelligence (AI) is no longer a futuristic concept—it’s here, reshaping industries, economies, and daily life. From chatbots answering customer queries to algorithms predicting global supply chain disruptions, AI’s capabilities are staggering. According to McKinsey, AI could contribute up to $13 trillion to the global economy by 2030. Yet, beneath this wave of innovation lies a growing unease: the ethical dilemmas of data privacy, algorithmic bias, and surveillance. How do we reconcile AI’s transformative potential with the need to protect individual rights? This blog dives deep into the challenges and solutions for building ethical AI systems that respect privacy without stifling progress.
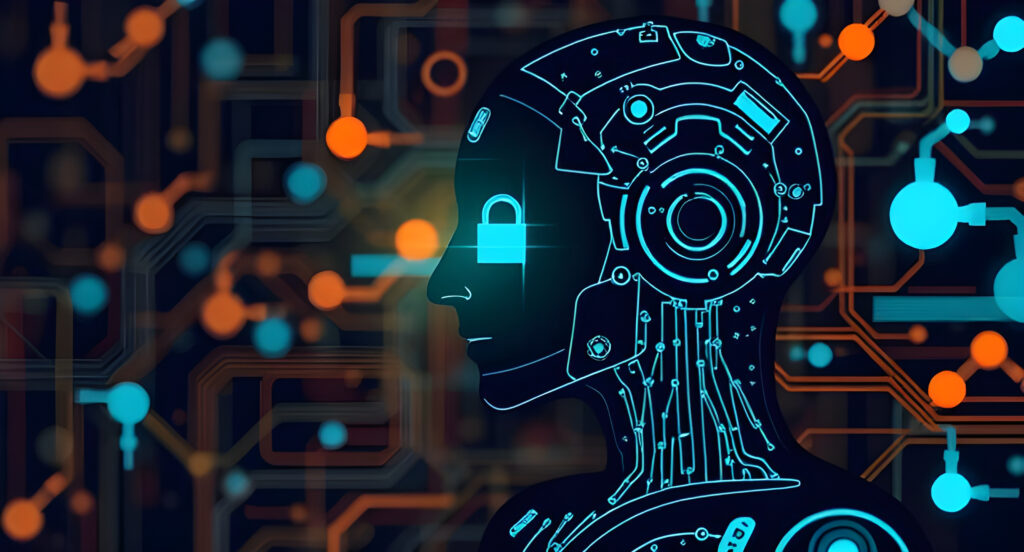
The Promise of AI Innovation: Transforming Industries
AI’s applications are vast, solving problems that once seemed insurmountable. Here’s how it’s driving change across sectors:
1. Healthcare: Saving Lives with Precision
AI is revolutionizing healthcare by enabling early diagnosis, personalized treatment, and drug discovery. For example:
- Cancer Detection: Google’s DeepMind developed an AI model that detects breast cancer in mammograms with 94% accuracy, outperforming human radiologists.
- Drug Development: During the COVID-19 pandemic, AI platforms like BenevolentAI identified existing drugs that could be repurposed to combat the virus, accelerating research timelines.
- Remote Monitoring: Wearables paired with AI algorithms track vital signs in real-time, alerting patients and doctors to anomalies like irregular heartbeats.
2. Finance: Security and Efficiency
AI is making financial systems safer and more inclusive:
- Fraud Detection: Mastercard’s AI-powered system analyzes transaction patterns to flag fraudulent activity in milliseconds, reducing false declines by 80%.
- Credit Scoring: Startups like Tala use alternative data (e.g., smartphone usage) to assess creditworthiness for underserved populations, expanding financial access.
- Algorithmic Trading: Hedge funds leverage AI to predict market trends, though this raises questions about fairness and market manipulation.
3. Sustainability: Fighting Climate Change
AI is a critical tool in the climate crisis:
- Energy Optimization: Google’s DeepMind reduced energy consumption in data centers by 40% using AI-driven cooling systems.
- Wildlife Conservation: AI-powered drones monitor deforestation and poaching in real-time, protecting endangered ecosystems.
These advancements highlight AI’s potential to drive societal progress. However, they also rely on massive datasets—often containing personal information—raising urgent privacy concerns.
Privacy Concerns in the Age of AI: The Risks of Unchecked Innovation
AI’s hunger for data is insatiable. To function effectively, systems require vast amounts of information, including sensitive details about individuals. Here are the key risks:
1. Data Exploitation and Consent
Many AI applications thrive on user data collected through opaque terms of service. For instance:
- Social Media: Platforms like Facebook and TikTok use AI to analyze user behavior, tailoring ads and content—often without explicit consent.
- Smart Devices: Voice assistants like Amazon’s Alexa record conversations, storing data that could be hacked or misused.
A 2023 Cisco survey found that 76% of consumers don’t trust organizations to use their data ethically. Yet, companies continue to prioritize data collection over transparency, creating a trust deficit.
2. Surveillance and Civil Liberties
Governments and corporations are deploying AI-powered surveillance tools at scale:
- Facial Recognition: China’s “Social Credit System” uses AI to monitor citizens’ behavior, affecting access to jobs and travel. In the U.S., cities like San Francisco have banned police use of facial recognition over racial bias concerns.
- Predictive Policing: Tools like PredPol claim to forecast crime hotspots but often target marginalized communities, reinforcing systemic inequities.
Such technologies risk normalizing a surveillance state, eroding privacy, and chilling free expression.
3. Algorithmic Bias and Discrimination
AI systems trained on biased data perpetuate inequality:
- Hiring Algorithms: Amazon scrapped an AI recruitment tool after discovering it downgraded resumes containing the word “women’s” (e.g., “women’s chess club”).
- Healthcare Disparities: A 2019 study found that an algorithm used in U.S. hospitals prioritized white patients over Black patients for care, as it relied on historical spending data (which reflected systemic inequities).
Without intervention, biased AI could deepen societal divides.
Ethical AI Frameworks: Bridging Innovation and Privacy
To address these challenges, stakeholders must adopt ethical AI frameworks that prioritize human rights. Key principles include:
1. Transparency and Explainability
Users deserve to know how AI decisions affect them. Explainable AI (XAI)—models that clarify their reasoning—is critical. For example:
- Healthcare: The EU’s General Data Protection Regulation (GDPR) grants patients the “right to explanation” for AI-driven diagnoses.
- Finance: Banks like HSBC use XAI to explain credit denials, building trust with customers.
2. Fairness and Bias Mitigation
Combating bias requires proactive measures:
- Diverse Datasets: IBM’s Diversity in Faces initiative created a dataset of 1 million facial images across varied ethnicities to reduce bias in facial analysis.
- Algorithmic Audits: Tools like Microsoft’s Fairlearn assess models for disparities, enabling developers to adjust outputs.
3. Accountability and Redress
Clear accountability structures are essential:
- AI Ethics Boards: Companies like Salesforce have internal boards to review high-risk AI projects.
- Legal Frameworks: The EU’s proposed AI Act categorizes systems by risk level, banning harmful uses (e.g., social scoring) and requiring audits for high-risk applications.
4. Data Minimization and Privacy-Preserving Techniques
Collecting less data reduces privacy risks:
- Federated Learning: Google uses this technique to train keyboard prediction models on user devices without transferring raw data to servers.
- Synthetic Data: Companies like Mostly AI generate artificial datasets that mimic real-world patterns, eliminating privacy concerns.
Collaborative Solutions: Governments, Tech, and Civil Society
Ethical AI requires collaboration across sectors:
1. Government Regulation
Policymakers must craft agile laws that protect privacy without stifling innovation:
- GDPR (EU): Fines up to 4% of global revenue for data misuse.
- California Consumer Privacy Act (CCPA): Grants residents the right to opt out of data sales.
- Global Standards: The OECD’s AI Principles promote inclusive growth and human-centered values across 42 countries.
2. Corporate Responsibility
Tech giants are adopting self-regulation:
- Google’s AI Principles: Prohibit AI use in weapons or surveillance that violates human rights.
- Microsoft’s Responsible AI Standard: Embeds ethics checks into product development cycles.
3. Academia and Advocacy
Research institutions and NGOs drive accountability:
- AI Now Institute: Publishes annual reports on AI’s societal impacts, urging bans on harmful technologies.
- Partnership on AI: A coalition of 100+ organizations (including Apple and ACLU) developing best practices for ethical AI.
Real-World Success Stories: Ethical AI in Action
Several organizations are proving that innovation and ethics can coexist:
1. Healthcare: Mayo Clinic’s Privacy-First Approach
Mayo Clinic uses AI to predict patient deterioration but anonymizes data and limits access to protect privacy. Their models are trained on aggregated datasets, ensuring individual identities remain hidden.
2. Technology: Apple’s Differential Privacy
Apple collects user data (e.g., typing habits) using differential privacy—adding “noise” to datasets to prevent identification. This allows improvements to services like Siri without compromising privacy.
3. Policy: Brazil’s LGPD
Brazil’s General Data Protection Law (LGPD), inspired by GDPR, mandates user consent for data collection and grants rights to access or delete personal information.
The Path Forward: A Blueprint for Balance
Critics argue that excessive regulation could slow innovation, but history shows that ethical guardrails can spur responsible growth. Strategies for the future include:
1. Privacy-by-Design
Embed privacy into AI development from the start:
- Homomorphic Encryption: Allows data analysis without decrypting sensitive information.
- Decentralized AI: Blockchain-based systems let users control their data while contributing to collective models.
2. Public Education and Empowerment
Educating users about data rights is crucial:
- Digital Literacy Programs: Schools and NGOs can teach individuals how to manage privacy settings and recognize AI risks.
- Transparency Reports: Companies should publish annual reports detailing data practices and bias audits.
3. Global Cooperation
AI’s challenges transcend borders:
- UN AI Advisory Body: Proposed by Secretary-General António Guterres to align global AI governance with human rights.
- Cross-Border Data Treaties: Agreements like the EU-U.S. Privacy Shield (under revision) must balance data flow and protection.
Conclusion: Building an Ethical AI Future
The stakes for ethical AI have never been higher. While the technology holds immense promise, its misuse could exacerbate inequality, erode privacy, and undermine democracy. By prioritizing transparency, fairness, and collaboration, we can create AI systems that reflect our shared values. Governments, corporations, and individuals all have roles to play—whether through regulation, innovation, or advocacy. The goal isn’t to halt progress but to ensure it serves humanity’s best interests. As we stand at this crossroads, the question isn’t whether AI will shape our future, but how. Let’s choose a path that balances innovation with integrity.
What steps do you think are most critical for balancing AI innovation and privacy? Share your thoughts in the comments below.