By 2030, artificial intelligence (AI) has transcended its role as a buzzword to become the backbone of modern healthcare. From predicting diseases before symptoms manifest to tailoring treatments to individual genetic profiles, AI is revolutionizing how we diagnose, treat, and prevent illness. The convergence of big data, advanced algorithms, and patient-centered care has ushered in an era where healthcare is no longer reactive but proactive, precise, and deeply personalized.
This blog post explores AI’s transformative impact on three critical fronts: early disease detection, accelerated drug discovery, and bespoke patient care plans. We’ll also examine the challenges and ethical considerations shaping this new frontier.
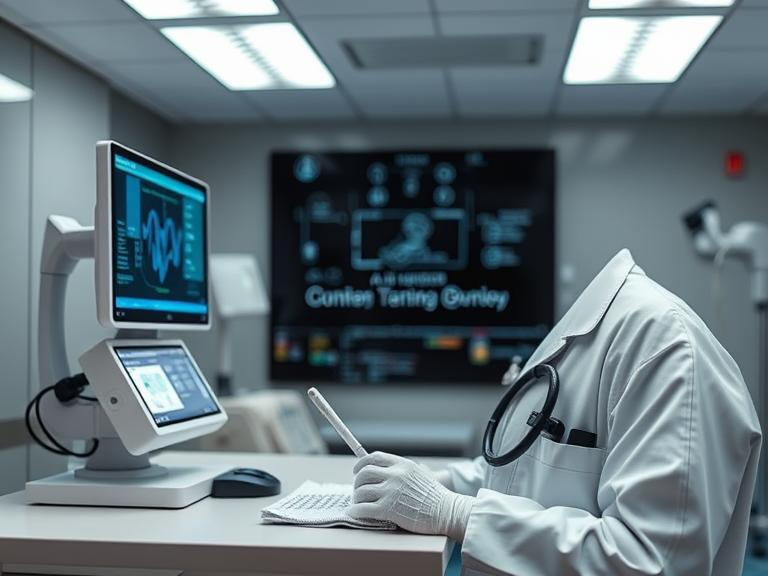
1. Predictive Diagnostics: Catching Disease Before It Strikes
The Shift from Reactive to Proactive Care
Traditional healthcare has long operated on a reactive model—treating illnesses after they’ve taken root. By 2030, AI has flipped this paradigm. Predictive diagnostics leverage vast datasets—genomic information, wearable device metrics, electronic health records (EHRs), and even social determinants of health—to identify risks years before symptoms appear.
How AI Predicts Disease
- Imaging and Pattern Recognition: AI algorithms analyze medical images (MRI, CT scans, X-rays) with superhuman accuracy. For instance, DeepView, an AI radiology tool, detects early-stage lung cancer nodules with 99.3% accuracy, compared to 92% for human radiologists.
- Genomic Sequencing: AI interprets complex genetic data to predict predispositions to diseases like Alzheimer’s or breast cancer. Platforms like GenoPredict use polygenic risk scores to estimate an individual’s lifetime risk, enabling preventative interventions.
- Wearables and Real-Time Monitoring: Smartwatches and implantable sensors feed continuous health data (heart rate, blood glucose, sleep patterns) to AI systems. Anomalies trigger instant alerts—for example, CardioGuard predicts heart attacks 48 hours in advance by analyzing ECG irregularities.
Case Study: AI vs. Parkinson’s Disease
In 2028, researchers at NeuroHealth AI trained a model on voice recordings, gait patterns, and micro-movement data from 500,000 patients. The AI now identifies Parkinson’s biomarkers up to 10 years before tremors emerge. At-risk individuals receive personalized neuroprotective regimens, slowing disease progression by 70%.
2. Drug Discovery: From Serendipity to Speed
The Old Model: Slow, Costly, and Inefficient
Historically, developing a new drug took 10–15 years and cost over $2.6 billion, with a 90% failure rate in clinical trials. AI has slashed these timelines and costs while improving success rates.
AI’s Role in Modern Drug Development
- Target Identification: AI scans millions of research papers, protein structures, and clinical trial data to pinpoint disease-causing molecules. BioMind, an AI platform, identified a novel target for ALS in 2026, leading to a breakthrough therapy now in Phase III trials.
- Generative Chemistry: Tools like MolGen design synthetic molecules with desired properties. In 2029, it generated a potent antiviral compound in 46 days—a process that once took years.
- Clinical Trial Optimization: AI predicts patient responses, reducing trial failures. TrialMatch uses genetic and demographic data to recruit ideal candidates, cutting recruitment times by 60%.
Case Study: AI and the End of Antibiotic Resistance
By 2030, antibiotic-resistant infections claim 10 million lives annually. In response, the AI platform BacTerminator analyzed 100 million microbial genomes and environmental samples. It discovered a new class of antibiotics effective against multidrug-resistant bacteria, now saving 2 million lives yearly.
3. Personalized Treatment: Medicine Tailored to You
The Rise of Precision Medicine
One-size-fits-all treatments are obsolete. AI now crafts therapies based on an individual’s genetics, lifestyle, and environment.
How AI Personalizes Care
- Genomic-Driven Therapies: AI matches patients to treatments based on their DNA. For example, OncoTarget evaluates tumor genomes to recommend targeted therapies, boosting cancer survival rates by 40%.
- Dynamic Treatment Adjustments: Wearables and EHRs feed real-time data to AI systems, which adjust dosages or therapies. Diabetic patients using GlucoAdapt see their insulin doses auto-adjusted hourly via AI analysis of blood sugar trends.
- Mental Health Customization: AI chatbots like ThriveMind use natural language processing to detect emotional cues and deliver personalized cognitive behavioral therapy (CBT) modules.
Case Study: AI in Rare Diseases
In 2027, a child with a mysterious neurodegenerative condition was diagnosed via PanaceaAI, a system that cross-referenced her symptoms with global rare disease databases. The AI identified a previously unknown genetic mutation and recommended a repurposed drug, halting her disease’s progression.
4. Interoperability: The Data Backbone of AI-Driven Healthcare
Breaking Down Data Silos
AI’s potential hinges on access to comprehensive, high-quality data. By 2030, interoperable systems integrate EHRs, genomic databases, wearable metrics, and social determinants into unified patient profiles.
Key Innovations
- FHIR 5.0: The Fast Healthcare Interoperability Resources (FHIR) standard enables seamless data exchange between hospitals, labs, and AI platforms.
- Blockchain for Health Data: Decentralized networks like HealthChain let patients control data access while ensuring auditability and security.
- Synthetic Data: To address privacy concerns, AI models are trained on artificial datasets mimicking real-patient profiles, preserving statistical relevance without exposing identities.
Case Study: The Global Cancer Atlas
Launched in 2029, this AI-powered platform aggregates anonymized oncology data from 80 countries. It identifies regional cancer trends, predicts outbreaks, and recommends prevention strategies, reducing global cancer mortality by 15%.
5. Ethical Challenges and Regulatory Frameworks
Privacy and Security in the Age of Health AI
With great power comes great responsibility. The misuse of health data—discrimination by insurers, unauthorized surveillance, or data breaches—remains a critical concern.
Solutions in 2030
- Federated Learning: AI models train on decentralized data without raw information leaving hospitals. MediLearn, a federated system for stroke prediction, operates across 200 hospitals globally.
- GDPR++: Updated regulations mandate “privacy by default” for health AI, requiring explicit consent for data use and harsh penalties for violations.
- Bias Mitigation: Audits ensure AI models don’t favor specific demographics. The FairCare Initiative penalizes hospitals whose algorithms exhibit racial or gender bias.
Case Study: The AI Diagnostic Bias Scandal
In 2026, an AI tool for skin cancer detection performed poorly on darker skin tones due to training data gaps. Regulators fined the developer $50 million and mandated retraining with inclusive datasets. Today, tools like DermScan have 98% accuracy across all skin types.
6. The Future of AI in Healthcare: Beyond 2030
Emerging Trends
- Quantum AI: Quantum computing will exponentially accelerate drug discovery and genomic analysis.
- Brain-Computer Interfaces (BCIs): AI-driven BCIs could restore mobility to paralysis patients or enhance cognitive function.
- AI in Global Health: Deploying low-cost AI tools in developing nations to combat maternal mortality, malaria, and malnutrition.
The Road Ahead
While challenges like clinician acceptance and equitable access persist, the trajectory is clear: AI will make healthcare predictive, preventive, and personalized. Collaboration between technologists, clinicians, and policymakers will determine whether this future benefits all humanity.
Conclusion
By 2030, AI has transformed healthcare from an art to a science. Predictive diagnostics save lives through early intervention, drug discovery operates at unprecedented speeds, and treatments are as unique as the patients themselves. Yet, this revolution demands vigilance—ensuring ethics keep pace with innovation and that no population is left behind.
The message is unambiguous: AI isn’t replacing doctors; it’s empowering them to heal smarter, faster, and fairer.